Shafae to Lead Water Quality Monitoring Efforts for Multimillion-Dollar Consortium
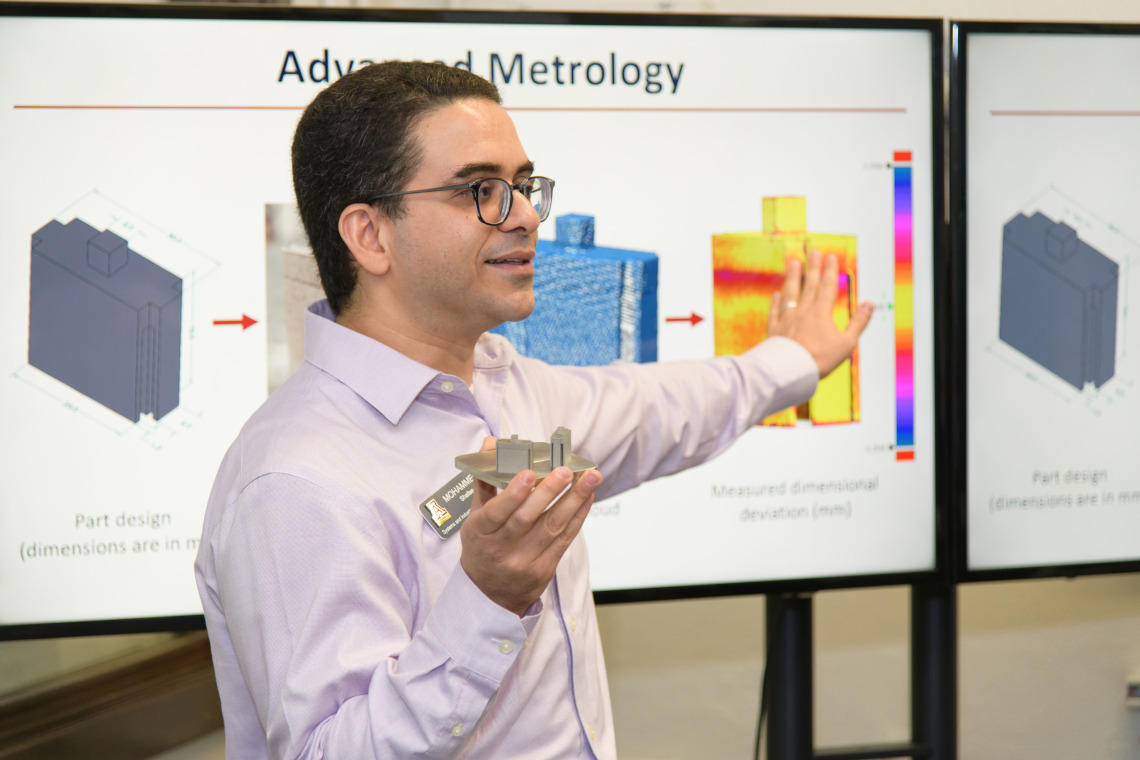
As traditional sources of water run low, concern around Arizona’s water supply is growing. The University of Arizona has received $4 million for phase one of a project to improve water security and water reuse methods in the arid southwestern United States, with more funds to come. Mohammed Shafae, an assistant professor in SIE, is leading the development of machine learning and artificial intelligence methods to monitor and maintain water quality.
With funding from the U.S. Army Engineer Research and Development Center's Construction Engineering Research Laboratory, the UA, the University of Southern California and the University of Nevada, Reno, are launching the Consortium for Potable Water Reuse.
"We are going to move towards autonomous and self-correcting water systems by using real-time measurements of process performance variables and correlating them to water quality metrics using machine learning,” Shafae said. “This will allow us to make timely decisions allowing fault detection, system self-correction, and performance forecasting – but most importantly, to enhance consumer confidence in reclaimed and reuse water."
The grant’s principal investigator is Andrea Achilli of chemical and environmental engineering, while other engineering co-investigators in the same department are Jim Farrell, Kerri Hickenbottom and Eduardo Saez.
The UA portion of the project aims to develop a decentralized model for water reuse methods by treating water at the neighborhood, or even the building level. This conserves resources because water doesn’t have to travel as far to be treated, and it also allows citizens a clearer understanding of where their water is coming from. But monitoring so many different treatment systems is a complex task, since each neighborhood or household will have different usage levels and treatment needs. These variations means a baseline recommendation such as system cleaning or changing a filter every three months isn’t a viable approach.
“That’s economically very important, because you don’t want the filter to go bad after two months, and you’re drinking bad water for a month,” said Shafae.”
Shafae describes the approach as a move from preventative maintenance to proactive maintenance. The water quality data gathered by sensors will be processed by machine learning and artificial intelligence methods developed by Shafae and his students in collaboration with the rest of the team. Recommendations for maintenance – whether it be changing a filter, dispatching a specialist, or potentially the system even correcting itself– will be based on this data, rather than on blanket rules that may not be suitable for all users and could be very costly.
“With these timely alerts, the system gets maintenance when it needs maintenance – whether it’s sooner or later than suggested by a predefined schedule,” Shafae said. “At the end of the day, we want to make sure we are delivering quality water in the most economical way, and I am excited my work can enable that.”