When
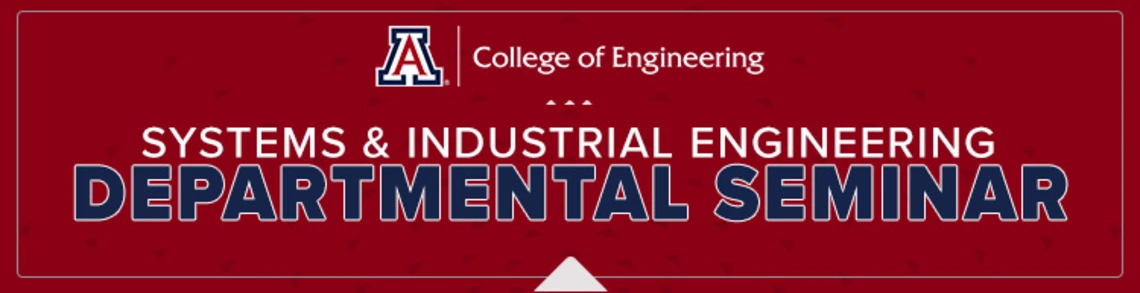
Thursday, September 12, 2024 - 2:00 p.m.
Eung-Joo Lee
Assistant Professor of Electrical & Computer Engineering
University of Arizona
"Deep Learning-Based Image Analysis on Resource-Constrained Systems"
ENGR Building, Room 301
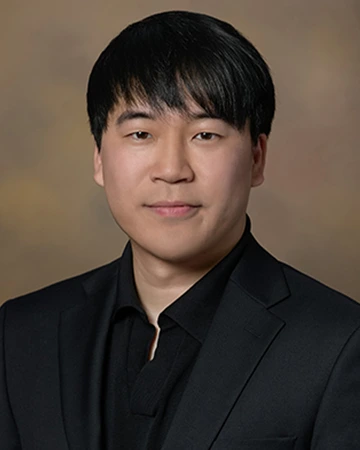
Abstract: In recent years, deep learning approaches have achieved high-end performance on various computer vision tasks. Especially, convolutional neural networks (CNNs) are extensively studied and utilized for image analysis. However, deploying deep learning on edge devices to solve practical computer vision tasks involves major challenges. This includes obtaining vast amounts of labeled training data for related downstream tasks, along with careful considerations regarding real-time requirements, memory consumption, and energy budgets for edge-based applications. In this presentation, I primarily focus on two essential factors for implementing deep learning in image analysis: (1) Ensuring the availability of sufficient labeled training data to achieve high accuracy and (2) Designing deep learning systems that leverage computationally efficient models suitable for practical scenarios. For the first topic, I will present data-centric approaches that aim to achieve model robustness through dataset generation. For the second, I will discuss the development of efficient deep neural network models tailored for reliable implementation on edge devices. Throughout my presentation, I will explore the application of embedded computer vision systems and medical imaging in resource- constrained environments.
Bio: Eung-Joo Lee is an assistant professor in the Department of Electrical and Computer Engineering at the University of Arizona. Before joining the University of Arizona, he was a postdoctoral fellow at MGH/Harvard Medical School and earned his PhD in electrical and computer engineering from the University of Maryland, College Park, where he also interned at the U.S. Army Research Laboratory. His research aims to develop deep learning systems that utilize computationally efficient models designed specifically for embedded computer vision systems and medical imaging applications. His focus extends to exploring the development of intelligent systems for a diverse range of applications, including unmanned vehicles, face analysis, hyperspectral imaging, and medical imaging application with multimodal learning.